报告主题:Tobit models for count time series
报 告 人:朱复康 教授
报告时间:2024年12月3日(周二)上午10:00-12:00
报告地点:腾讯会议(会议号:116181382)
报告摘要: Several models for count time series have been developed during the last decades, often inspired by traditional autoregressive moving average (ARMA) models for real-valued time series, including integer-valued ARMA (INARMA) and integer-valued generalized autoregressive conditional heteroscedasticity (INGARCH) models. To achieve negative ACF values within the class of INGARCH models, log and softplus link functions are suggested in the literature, where the softplus approach leads to conditional linearity in good approximation. A Skellam--Tobit INGARCH model for unbounded counts is studied in detail, including stationarity, approximate computation of moments, maximum likelihood and censored least absolute deviations estimation for unknown parameters and corresponding simulations. Extensions of the Tobit approach to other situations are also discussed, including underlying discrete distributions, INAR models, and bounded counts. Three real-data examples are considered to illustrate the usefulness of the new approach.
报告人简介:朱复康,吉林大学44118太阳成城集团教授、博士生导师,吉林国家应用数学中心副主任、院长助理、概率统计与数据科学系主任。主要从事时间序列分析和金融统计的研究,主持国家自然科学基金面上项目3项和青年基金1项。曾获得教育部自然科学奖二等奖、吉林省科学技术奖二等奖、长春市有突出贡献专家等奖励,连续两年(2022-2023)入选美国斯坦福大学发布的全球前2%顶尖科学家榜单。现任中国现场统计研究会、全国工业统计学教学研究会、中国数学会概率统计分会等学会的理事或常务理事。在Annals of Applied Statistics、中国科学-数学等期刊上发表论文多篇,现任Statistical Papers等期刊的Associate Editor,是JASA、JRSSB、JBES、AoAS等80余个SCI期刊的匿名审稿人。
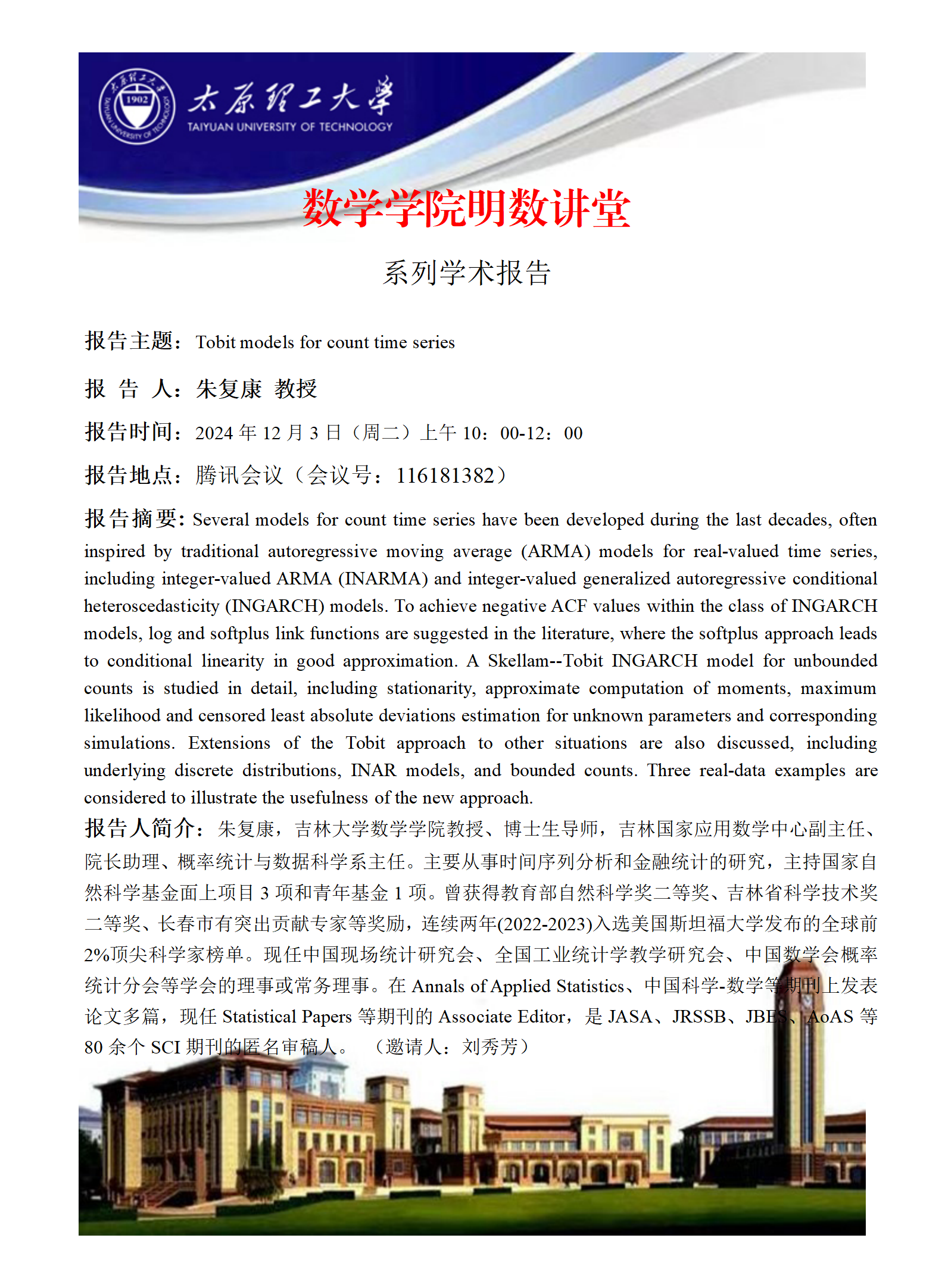